
The MIHRA Bio-Data Harmonization Core addresses the essential need for global pooling of biological and case information in rare diseases in order to elucidate critical patterns of disease typing, diagnosis, severity, complications, and potential treatments.
MIHRA Bio-Data Harmonization Core is a proactively involved in MIHRA’s public private partnership (PPP) research efforts to ensure sound clinical trial design and implementation. MIHRA’s PPP requires close working interactions and decision-making with industry, patient partners & organizations, partner research organizations and regulatory agencies to establish shared priorities and use of existing trial data to efficiently & holistically improve clinical trial readiness across myositis diseases. This includes dedication to improving reliability of endpoints and other measures of disease response, criteria for disease activity and disease status such as ‘remission‘ and ‘refractory‘.
MIHRA Bio-Data Harmonization Core also houses MIHRA’s Analytic Sub-Core of statisticians, data analysts & epidemiologists that represent pan-diversity in type of expertise in both quantitative, qualitative, bio-informatics and machine learning applications in rare diseases research.
MIHRA works to ensure that collaborative community research projects in myositis-related diseases have access to high level analytic expertise and software in both designing studies and analyzing study results. MIHRA also proactively supports mentorship in the analytical applications in rare diseases for rising analytic professionals as well as to fortify knowledge for the clinical researcher.
Sub-Cores for Bio-Data Standardization, Feasibility & Quality
For the world community of myositis researchers to collaborate with shared clinical information and specimens, and produce results that are meaningful, reliable and interpretable, the elements need to be the same as well as collected and processed with agreed quality standards. In addition, collecting clinical data and biospecimens occur in the busy and pressured clinic relying upon the well-meaning & overworked clinician.
So for successful collaboration that contributes a reliable volume of cases with the highest quality possible, the collection and processing procedures need to be done in ways that are as easy as possible for the clinician.
Current and future projects of each of these sub-cores seek to establish:
- global community consensus (which includes patients) on feasible, accessible and compliant data and specimen collection
- standards of bio-specimen processing and testing that preserve sample potency and best alignment with the clinical picture and disease severity.
Bio-Specimen Sub-Core
works to establish standardized protocols and infrastructure to ensure high quality research samples for clinical projects. This is in contrast to the other important efforts such as Accelerating Medicines Partnership (AMP) Autoimmune & Immune-Mediated Disease Program (AIM), which focuses on utilizing biospecimen samples to understand cellular and molecular interactions leading to inflammation and autoimmunity.
MIHRA ultimate goals are to identify procedural techniques that retain the highest disease activity profiles that most accurately reflect disease activity states to inform clinically meaningful outcomes when coupled with clinical data Learn more..
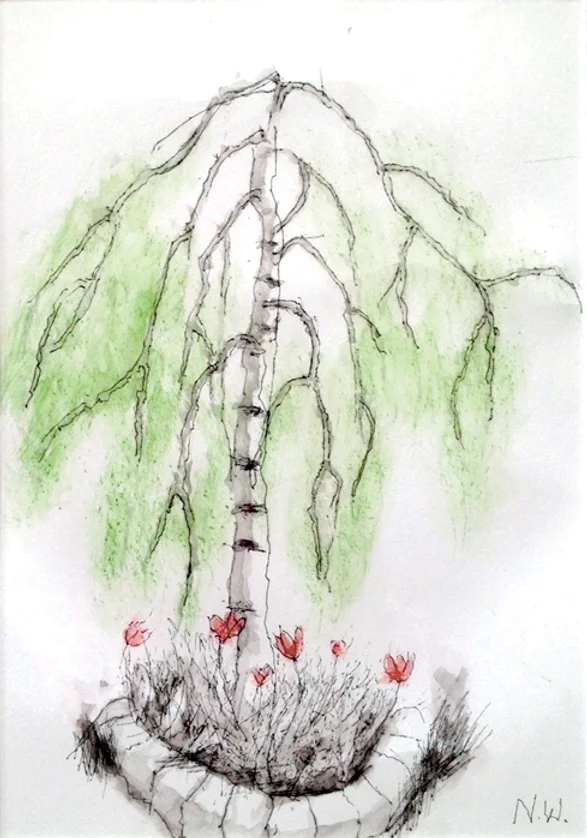
Clinical Data Sub-Core
works to determine the community’s (including patients’) priorities, preferences, and perceived feasibility on collecting common data elements (CDEs) to develop minimal and optimal data sets. This includes investigations into barriers to longitudinal data collection across diverse clinical setting and identify outcome measures that are actually being utilized in clinical practice and, if standard measures are not being used, to explore the root cause(s).
Global legacy data from divergent databases by mapping to CDEs and common data models such as Observational Medical Outcomes Partnership (OMOP) will be able to be merged with current prospective collections in a common, sustainable, and feasible global database.